Abstract
One key feature of Bluetooth low energy (BLE) beacons is the received signal strength (RSS), which can be used toestimate the distance between any Bluetooth-compatible receiver (e.g., smartphone, tablet, etc.) and fixed deployed beacon. AlthoughRSS can be measured easily with commonly available smart devices, the measurements are unreliable, in which general estimationmodels are not robust to different hardware and settings for real deployed beacon networks. Furthermore, the lack of consideration forobject mobility in these models undermines its practicality. Motivated by the above limitations, this paper proposes a novel distanceclassifier, d-Classifier, to classify the distance with a feature vector constructed with factors such as hardware type and deploymentenvironment to improve the robustness. Moreover, comprehensive experiments related to mobility are conducted to study therelationship between packet receiving rate and estimation accuracy. Improved performance can be achieved by providing extra mobilityinformation with a list of RSS values during estimation. The proposed classifier is validated with an extensive dataset that includes over200kdata collected from real beacon networks. Overall, our proposed d-Classifier achieves a significant performance gain,>25%accuracy improvement, over its prior arts.
Beacon dataset for distance estimation
To improve the robustness of the proposed method, this paper uses a large-scale beacon dataset with more than 200k records for justification and performance evaluation. The dataset is created by our team through different BLE beacons related projects. A mobile application is developed for collect- ing data and storing it in the cloud. Our team is continuously updating the dataset; however, the latest statistical information of the dataset at the time of writing is listed as follows:

The labels of the dataset are listed as follows:
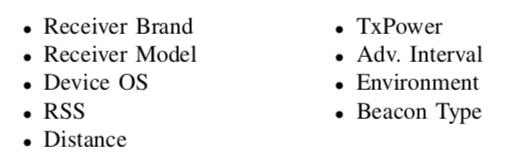
Some deploy environment examples with the corresponding environment type are shown in the following:
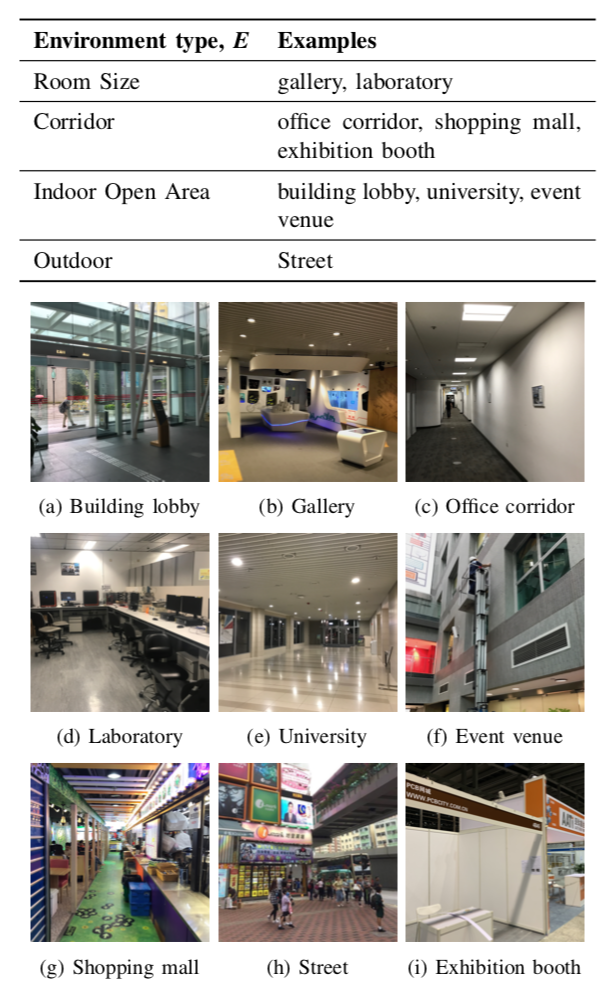
Download
The dataset is available for download here: .CSV file
Experiment Tools & Video
The mobile application can be access here
Related Paper
Ching Hong Lam, Pai Chet Ng and James She, "Improved Distance Estimation with BLE Beacon using Kalman Filter and SVM," 2018 IEEE International Conference on Communications .pdf, IEEE Xplore
Ching Hong Lam and James She, "Distance Estimation on Moving Object using BLE Beacon," 2019 IEEE International Conference on Wireless and Mobile Computing, Networking and Communications .pdf, IEEE Xplore
K. E. Jeon, J. She, P. Soonsawad and P. C. Ng, "BLE Beacons for Internet of Things Applications: Survey, Challenges and Opportunities," in IEEE Internet of Things Journal .pdf, IEEE Xplore